Large-Scale CARLA Scenario Design
A dataset covering 10 intersections with diverse geometries, traffic densities, and environmental conditions for multi-modal sensor placement evaluation.
Overview
Infra-Set is a large-scale dataset designed to support multi-modal infrastructure sensor placement evaluation in autonomous driving scenarios. It covers 10 intersections in various CARLA towns, each selected to represent diverse geometries, traffic densities, and ambient conditions, including varying lighting and weather conditions. The dataset provides a robust foundation for cooperative perception research by incorporating multi-agent, multi-modal sensor configurations.
Key Features
- Diverse Intersection Selection
- 10 intersections from CARLA towns 3, 4, 5, 6, 7, and 10
- Includes 4 four-way intersections, 2 T-intersections, 1 bridge-entry intersection, 1 roundabout, 1 five-way intersection, and 1 highway-entry T-intersection
- Categorized into urban, highway, and rural environments
- Large-Scale Dataset
- Contains 144,000 scenario frames with 2.6TB of data
- Includes camera and LiDAR data from at least nine sensor placements
- Multi-Agent Object Tracking
- Covers four primary object categories: cars, pedestrians, cyclists, and trucks
- Analyzes object density and movement across various traffic conditions
- Comparison with Existing Datasets
- Outperforms other cooperative perception datasets in number of intersections, infrastructure complexity, and sensor configurations
- The only dataset designed specifically for heterogeneous sensor placement research
Intersection Selection
To ensure diversity in the dataset, we carefully selected intersections based on real-world traffic flow and geometric variations. The intersections are categorized as:
- Large intersections (4)
- Medium-sized intersections (4)
- Small intersections (2)
They are further classified by environment type:
- Urban intersections (6)
- Highway intersections (3)
- Rural intersection (1)
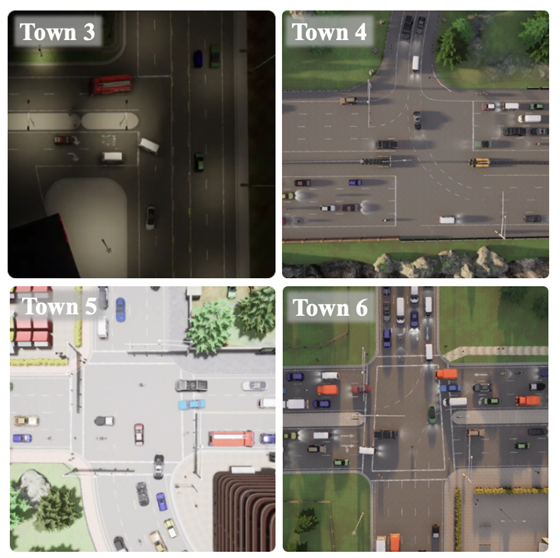
Dataset Structure & Size
The dataset consists of:
- 144,000 scenario frames
- Data from 9+ unique sensor configurations, including camera placements (Cam-c, Cam-d1, Cam-d2, Cam-d3) and LiDAR placements (L-c, L-d1, L-d2)
- 2.6TB total data volume
Data Analysis
The dataset covers three distinct traffic flow densities:
- High-density (~60 objects per scene)
- Medium-density (~40 objects per scene)
- Low-density (~20 objects per scene)
Each scene includes a balanced distribution of object types, ensuring representation across autonomous driving environments.
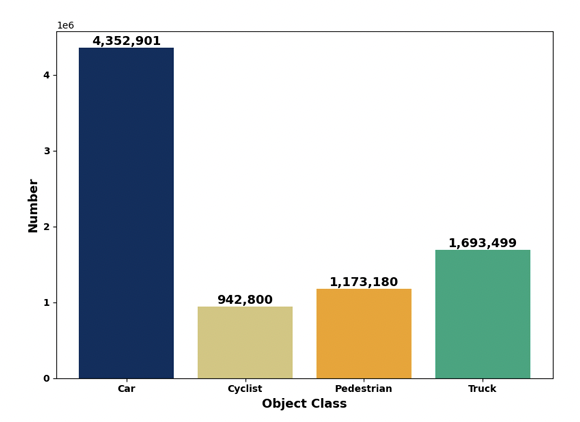
Comparative Analysis with Other Datasets
Infra-Set significantly outperforms existing cooperative perception datasets in:
- Number of intersections
- Total infrastructure coverage
- Data volume and diversity
Dataset | Year | Cooperation Mode | RGBs | LiDARs | Infrastructure Support | Task Type |
---|---|---|---|---|---|---|
OPV2V | 2022 | V2V | 44k | 11k | 4 | 3D |
V2X-Sim | 2022 | V2X | 60k | 10k | 1 | 3D |
V2XSet | 2022 | V2X | 44k | 11k | 3 | 3D |
DAIR-V2X | 2022 | V2X | 39k | 39k | 4 | 3D |
V2V4Real | 2023 | V2V | 40k | 20k | 2 | 3D |
V2X-Real | 2024 | V2X | 171k | 33k | 2 | 3D |
Rcooper | 2024 | Infra | 50k | 30k | 2/4 | 3D |
Infra-Set (Ours) | 2025 | Infra | 3,546k | 1,008k | 2–8 | 3D |
Infra-Set is the only dataset that supports heterogeneous sensor placement research, making it a crucial benchmark for infrastructure-based cooperative perception.
Visualization & Insights
We provide visualizations of dataset samples, illustrating:
- Object distribution across different categories (cars, pedestrians, cyclists, trucks)
- Traffic flow analysis across selected intersections
- Bounding box distributions for object detection tasks
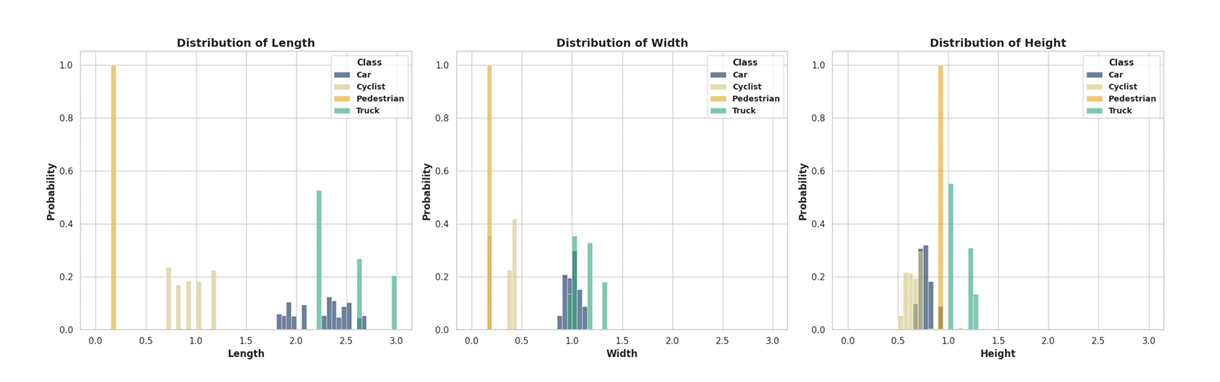
Conclusion
Infra-Set is a scalable, diverse, and high-fidelity dataset tailored for multi-modal infrastructure sensor placement research. Its unparalleled data volume, intersection diversity, and real-world traffic flow representation make it a valuable resource for advancing cooperative perception and intelligent intersection design in autonomous driving.